This report presents a practical approach to stacked generalization in surrogate model based optimization. It exemplifies the integration of stacking methods into the surrogate model building process.
First, a brief overview of the current state in surrogate model based optimization is presented. Stacked generalization is introduced as a promising ensemble surrogate modeling approach. Then two examples (the first is based on a real world application and the second on a set of artificial test functions) are presented. These examples clearly illustrate two properties of stacked generalization:
(i) combining information from two poor performing models can result in a good performing model and
(ii) even if the ensemble contains a good performing model, combining its information with information from poor performing models results in a relatively small performance decrease only.
The paper can be downloaded from https://cos.bibl.th-koeln.de/frontdoor/index/index/docId/375
Free Preprint: Stacked Generalization of Surrogate Models – A Practical Approach
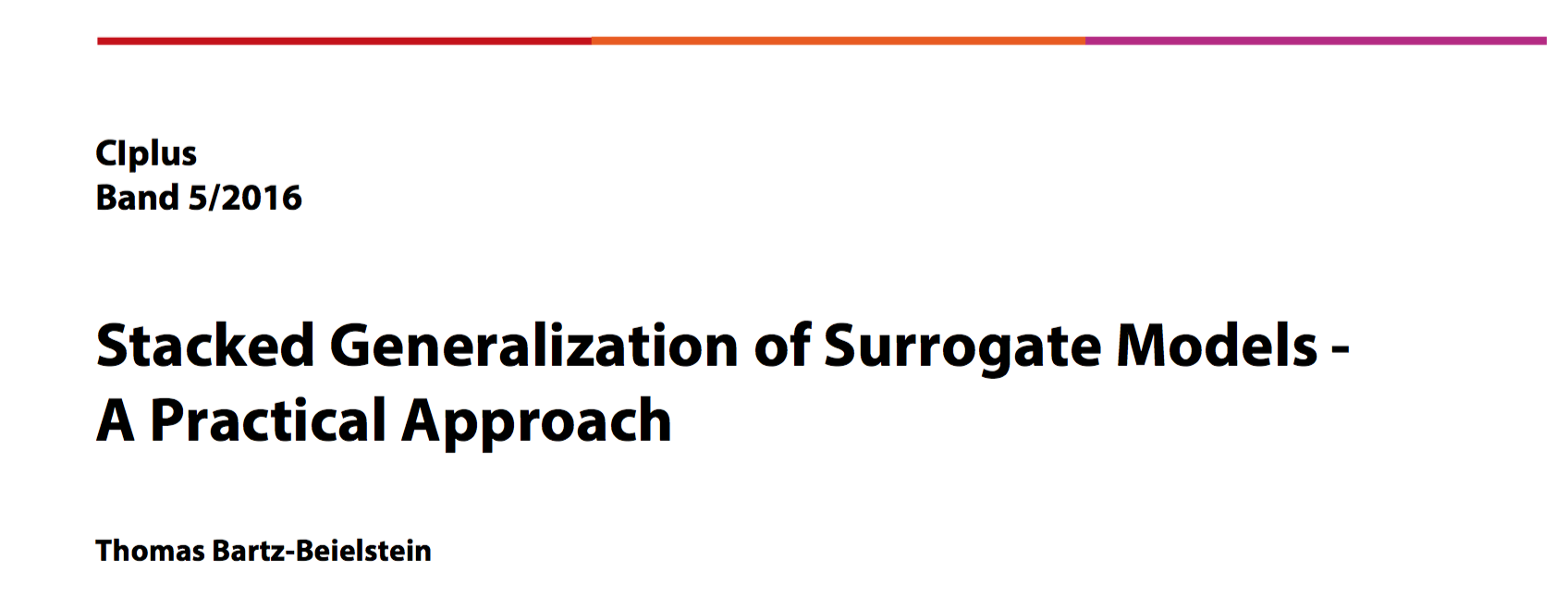